Communications and Signal Processing Seminar
Sequential Decision Making: How Much Adaptivity Is Needed Anyways?
This event is free and open to the publicAdd to Google Calendar
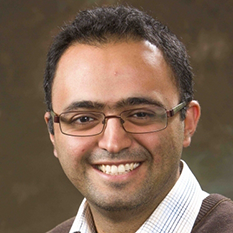
ABSTRACT: Adaptive stochastic optimization under partial observability is one of the fundamental challenges in artificial intelligence and machine learning with a wide range of applications, including active learning, optimal experimental design, interactive recommendations, viral marketing, Wikipedia link prediction, and perception in robotics, to name a few. In such problems, one needs to adaptively make a sequence of decisions while taking into account the stochastic observations collected in previous rounds. For instance, in active learning, the goal is to learn a classifier by carefully requesting as few labels as possible from a set of unlabeled data points. Similarly, in experimental design, a practitioner may conduct a series of tests in order to reach a conclusion. Even though it is possible to determine all the selections ahead of time before any observations take place (e.g., select all the data points at once or conduct all the medical tests simultaneously), so-called a priori selection, it is more efficient to consider a fully adaptive procedure that exploits the information obtained from past selections in order to make a new selection. In this talk, we introduce semi-adaptive policies, for a wide range of decision-making problems, that enjoy the power of fully sequential procedures while performing exponentially fewer adaptive rounds.
BIO: Amin Karbasi is currently an associate professor of Electrical Engineering, Computer Science, and Statistics & Data Science at Yale University. He is also a research scientist at Google NY. He has been the recipient of the Bell Labs Prize, National Science Foundation (NSF) Career Award, Office of Naval Research (ONR) Young Investigator Award, Air Force Office of Scientific Research (AFOSR) Young Investigator Award, DARPA Young Faculty Award, National Academy of Engineering Grainger Award, Amazon Research Award, Google Faculty Research Award, Microsoft Azure Research Award, Simons Research Fellowship, and ETH Research Fellowship. His work on machine learning, statistics, and computational neuroscience has received awards at several premier conferences and journals, including Medical Image Computing and Computer-Assisted Interventions Conference (MICCAI), Facebook-MAIN award from AI-Neuroscience symposium, International Conference on Artificial Intelligence and Statistics (AISTAT), IEEE Communications Society Data Storage, International Conference on Acoustics, Speech, and Signal Processing (ICASSP), ACM SIGMETRICS, and IEEE International Symposium on Information Theory (ISIT). His Ph.D. work received the Patrick Denantes Memorial Prize for the best doctoral thesis from the School of Computer and Communication Sciences at EPFL, Switzerland.
Join Zoom Meeting https://umich.zoom.us/j/91771072666
Meeting ID: 917 7107 2666
Passcode: XXXXXX (Will be sent via e-mail to attendees)
Zoom Passcode information is also available upon request to Katherine Godwin ([email protected]).