Communications and Signal Processing Seminar
Robustness to Incorrect Models and Approximations in Stochastic Control
This event is free and open to the publicAdd to Google Calendar
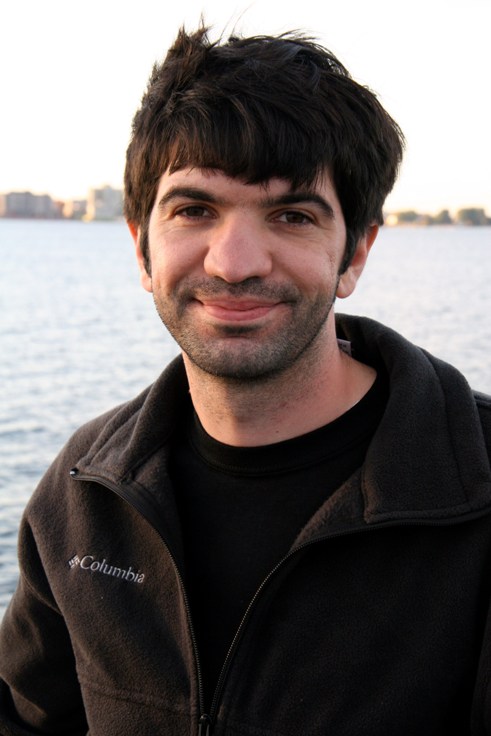
Abstract In stochastic control, typically, an ideal model is assumed or an estimate model is learned, and the control design is based on this model, raising the problem of performance loss due to the mismatch between the assumed model and the actual system. Even when a correct model is available, computation constraints may dictate the use of approximation methods. In this talk, we will view approximations and robustness under a unified theme through a topological approach involving spaces of probability measures. We will initially consider robustness to approximations for stochastic control with standard Borel spaces and present conditions under which finite models obtained through quantization of the state and action sets can be used to construct approximately optimal policies. We will establish that weak continuity of the transition kernel in the state and action is sufficient for the convergence of finite approximations. We will also extend our analysis to partially observed models and non-linear filtering processes where one can construct a sequence of finite models whose solutions constructively converge to the optimal cost; a byproduct of this analysis is a new weak Feller characterization for non-linear filter dynamics and through such weak continuity a rigorous approximation result follows. We will then investigate robustness to more general modeling errors and study the mismatch loss of optimal control policies designed for incorrect models applied to the true system, as the incorrect model approaches the true model. We show that continuity and robustness cannot be established under weak and setwise convergences of transition kernels in general, but that the expected induced cost is robust under total variation convergence. By imposing further assumptions (such as continuous convergence of transition kernels or total variation continuity on a measurement channel), we show that the optimal cost can be made continuous and robust under weak convergence of the transition kernels as well.
These results entail positive implications on empirical learning in data-driven stochastic control since often system models are learned through empirical data where typically (almost surely) weak convergence criterion applies but stronger convergence criteria do not. (joint work with Ali Kara, Naci Saldi and Tamas Linder)
Biography
Serdar Yuksel is an associate professor in the Department of Mathematics and Statistics at Queen’s University, Canada. He received his undergraduate degree in electrical and electronics engineering from Bilkent University (2001), and the MSc and PhD (2006) degrees in Electrical and Computer Engineering from the University of Illinois at Urbana-Champaign. After a post-doctoral position at Yale University, he has been with Queen’s University since 2007. His research interests are on stochastic control, decentralized control, information theory and probability. He has co-authored two books on networked control and stochastic control, received several awards including the 2013 CAIMS/PIMS early career award in applied mathematics, and has been serving on the editorial boards of several journals.