Control Seminar
Fast Online Reinforcement Learning Control using State-Space Dimensionality Reduction
Add to Google Calendar
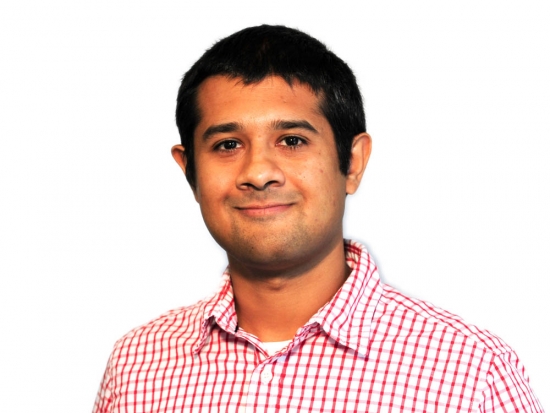
Abstract
Reinforcement Learning (RL) is an effective way of designing model-free linear quadratic regulator (LQR) controller for linear time-invariant (LTI) networks with unknown state-space models. However, when the network size is large, conventional RL can result in unacceptably long learning times. In this talk I will present some recent results on how we can resolve this problem by developing an alternative approach that combines dimensionality reduction with RL theory. The approach is to construct a compressed state vector by projecting the measured state through a projective matrix, which is constructed offline using probing signals. This matrix can be viewed as an empirical controllability Gramian that captures the level of redundancy in the open-loop network model. Next, a RL-controller is learned using the compressed state instead of the original state such that the resultant cost is close to the optimal LQR cost. The talk will end by highlighting the potential use of this method for widearea oscillation damping control of large-scale electric power systems.
Biography
Aranya Chakrabortty received his PhD degree from Rensselaer Polytechnic Institute, Troy, New York, in 2008 in electrical engineering. From 2008 to 2009, he was a postdoctoral research associate at the University of Washington, Seattle. From 2009 to 2010, he was an assistant professor of electrical and computer engineering at Texas Tech University, in Lubbock. Since 2010, he has been a faculty member of the Electrical and Computer Engineering Department at North Carolina State University, Raleigh, where he is currently an associate professor, a university faculty scholar, and also affiliated to the NSF FREEDM Systems Center. His research interests are in all branches of control theory with applications to electric power systems, and more recently in exploring new research topics at the intersection of control and reinforcement learning. He currently serves as an editor for IEEE Transactions on Power Systems and IEEE Transactions on Control System Technology. He received the NSF CAREER Award in 2011.