Dissertation Defense
Modeling and Parameter Identification for Condition Monitoring of Permanent Magnet Synchronous Machine Drive Systems
This event is free and open to the publicAdd to Google Calendar
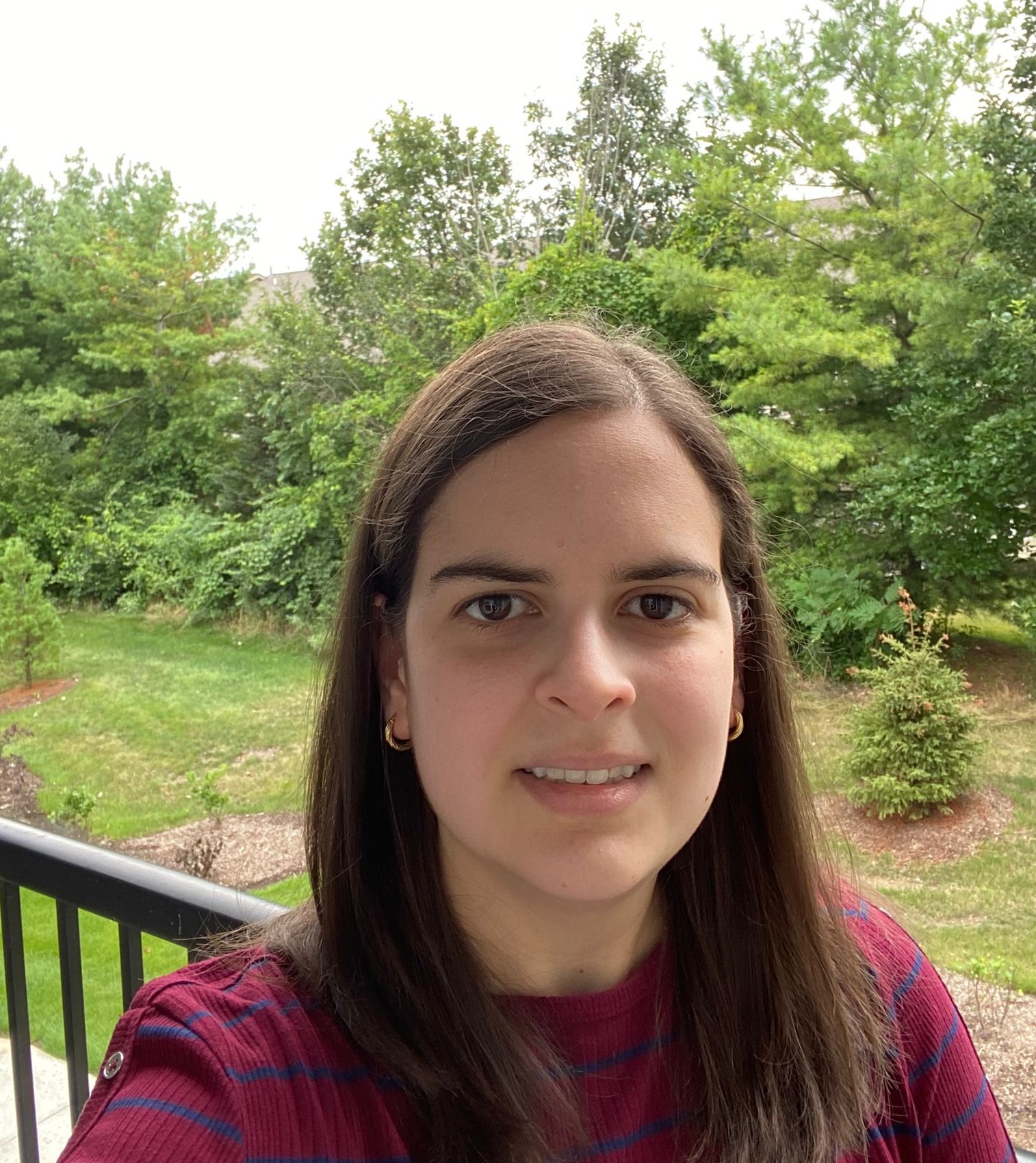
Permanent Magnet Synchronous Machines (PMSMs) have been preferred for high-performance applications given their high torque density, high power density, and potential for precise control and high efficiency over a wide operating range. However, PMSMs can experience faults, compromising the system performance and safety. Fault diagnosis and condition monitoring techniques aim to recognize abnormal conditions and track the health status during operation. Online parameter identification provides continuous estimates, which can be leveraged to monitor the machine’s health. However, some parameters vary with operating conditions, and the faults produce dynamic imbalances. Moreover, accurate estimation requires persistently exciting inputs, which may conflict with the control objectives.
This dissertation presents research that seeks to address open issues regarding the application of parameter identification to fault diagnosis and condition monitoring of Surface-Mount Permanent Magnet (SMPM) machines. Specifically, we propose models and parameter identification strategies for demagnetization, eccentricity, and inter-turn short conditions. The models characterize the distinctive dynamic features of SMPM machines under different fault conditions. The parameter identification techniques are designed to ensure accurate estimation while still satisfying control objectives. Finally, a parameter identification strategy for comprehensive fault detection is formulated by incorporating the estimators for demagnetization, eccentricity, and inter-turn short into a single strategy.
Co-Chairs: Professor Heath Hofmann & Professor Jing Sun