Communications and Signal Processing Seminar
Low-rank Matrix Completion under Monotonic Transformation
Add to Google Calendar
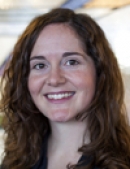
Most recent results in matrix completion assume that the matrix under consideration is low-rank or that the columns are in a union of low-rank subspaces. In real-world settings, however, the linear structure underlying these models is distorted by a (typically unknown) nonlinear transformation. This presentation will discuss our work that addresses the challenge of matrix completion in the face of such nonlinearities. Given a few observations of a matrix that are obtained by applying a Lipschitz, monotonic function to a low rank matrix, our task is to estimate the remaining unobserved entries. We propose a novel matrix completion method that alternates between low-rank matrix estimation and monotonic function estimation to estimate the missing matrix elements. Mean squared error bounds provide insight into how well the matrix can be estimated based on the size, rank of the matrix and properties of the nonlinear transformation. Empirical results on synthetic and real-world datasets demonstrate the competitiveness of the proposed approach. This is work with Ravi Ganti and Rebecca Willett at UW-Madison.
I am an Assistant Professor in the Electrical Engineering and Computer Science department at the University of Michigan, Ann Arbor.
My research projects are in statistical signal processing and estimation, particularly dealing with large and messy data. I have worked in the areas of online algorithms, compressed sensing and matrix completion, network inference, and sensor networks. My interests are theoretical, however, my favorite mathematical problems are motivated by fascinating and important engineering problems.