Dissertation Defense
Distributionally Robust Optimal Power Flow with Strengthened Ambiguity Sets
Add to Google Calendar
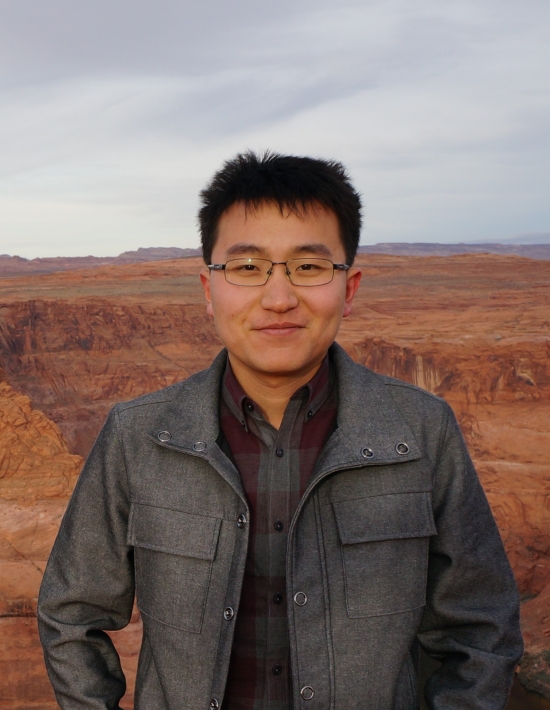
Abstract:
Uncertainties associated with renewable generation and load consumption have been a major source of risk to power systems. Different stochastic optimal power flow formulations have been proposed to safely incorporate these uncertainties using chance constraints, but conventional approaches either provide overly conservative results or rely on accurate estimates of uncertainty distributions that are rarely known. Solutions with poor estimates can result in low system reliability. To overcome this challenge, researchers have explored the use of distributionally robust optimization applied to optimal power flow, in which a family of distributions within a pre-defined ambiguity set is considered. Ambiguity sets are typically based on moment information (e.g., mean and covariance) of the random variables; however, specifying additional statistical characteristics reduces conservatism of the worst-case distribution. Hence, we develop approaches to strengthen the moment-based ambiguity sets by additionally incorporating structural information, such as unimodality and log-concavity, which are satisfied by most practical uncertainties. To efficiently solve the resulting stochastic optimization problems, we derive different reformulations and approximations and develop specific algorithms that guarantee global optimality. Using a variety of test networks, we observe a significant performance improvement compared with common approaches and obtain solutions with better trade-offs regarding reliability and objective costs.