Communications and Signal Processing Seminar
Data-Analytic Opportunities and Challenges in Solar Eruption Forecasting
This event is free and open to the publicAdd to Google Calendar
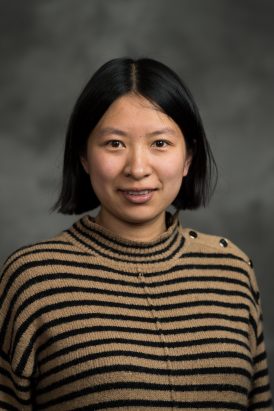
Abstract: In recent years, there has been a growing awareness of space weather impacts on critical infrastructure in the civilian, commercial, and military sectors. The interest will continue to grow as we gain a better understanding of the physical processes of the Sun and their effects here on Earth and in space itself. Among all space weather events, the solar flare is a relatively intense, localized emission of electromagnetic radiation in the Sun’s atmosphere. Flares occur in active regions and are often, but not always, accompanied by coronal mass ejections, solar particle events, and other eruptive solar phenomena. In recent years, machine learning models have been employed to forecast solar eruptions, including strong solar flare events.
In this talk, I will discuss data-analytic opportunities and challenges in solar eruption forecasting, given the availability of massive solar imaging data and other relevant data products. Scientifically, it is essential to (a) identify the solar active regions that have high potential to erupt in an automated fashion, (b) extract features from observed solar images using principled statistical algorithms, (c), most importantly, provide a probabilistic forecast for the eruption time, magnitude and magnetic field configuration, and (d) facilitate new understandings of the mechanism/physics of disruptive space weather events. In particular, I will present one of our novel statistical models, the Tensor Gaussian Process with Contraction, for solar flare forecasting, combining data from various types and sources. I will conclude with ongoing and future work on operational solar eruption forecasting.
Please find relevant papers and presentation slides on my website here: https://yangchenfunstatistics.github.io/yangchen.github.io//
BIO: Yang Chen is currently an Assistant Professor at the Department of Statistics at the University of Michigan and a research Assistant Professor at Michigan Institute for Data Science (MIDAS). Yang Chen received her Ph.D. (2017). She received her B.A. in Mathematics and Applied Mathematics from the University of Science and Technology of China (2011). Research interests include Bayesian modeling and computation, Hidden Markov models and general finite mixture models, Applied statistics and machine learning in astronomy and space science, Statistical methods for large-scale imaging data, Spatial-temporal data analysis, Uncertainty quantification for complex models
*** The event will take place in a hybrid format. The location for in-person attendance will be room 1200 EECS. Attendance will also be available via Zoom.
Join Zoom Meeting: https://umich.zoom.us/j/91414297851
Meeting ID: 914 1429 7851
Passcode: XXX (Will be sent via email to attendees)
Zoom Passcode information is available upon request to Shelly Feldkamp ([email protected]).