Dissertation Defense
Video Understanding with Minimal Human Supervision
This event is free and open to the publicAdd to Google Calendar
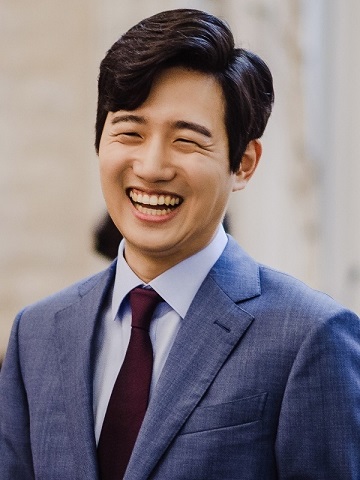
Passcode: 090203
There has been historic progress in the field of image understanding over the past few years. Deep learning models have come close to–or in several cases even surpassed–human performance in diverse tasks such as image classification, object detection, and instance segmentation. A large factor of this improvement has been the creation and sharing of large-scale datasets with a massive amount of manually-collected annotations. However, collecting annotations for video datasets compared to image datasets with similar scales is more challenging, time-consuming, and expensive, since a video can have an arbitrarily large number of frames. For example, collecting an autonomous driving dataset requires a lot of human annotators to draw the bounding boxes of all the pedestrians and vehicles for every frame of a video.
In this dissertation, we address video understanding problems with minimal human supervision. Specifically, we investigate two different approaches to reduce the need for expensive and labor-intensive annotations. The first is to improve the performance of video understanding models by only using easy-to-collect and less labor-intensive annotations. The second is to build weakly-supervised models, which only use partially-annotated videos. Both approaches can effectively alleviate the burden of collecting expensive manually-collected annotations.
Co-Chairs: Professors Jason J. Corso & Laura Balzano