Dissertation Defense
Strategic Signaling under Higher-order Inference
This event is free and open to the publicAdd to Google Calendar
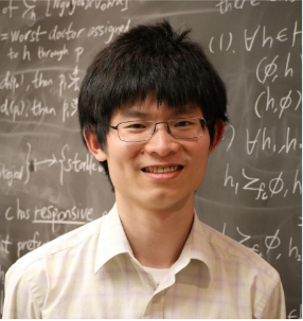
Passcode: 0inference
Inferring the information structure of other agents is necessary to derive optimal mechanisms/signaling strategies in games with communications. However, in many real-world problems such as fake news detection, drug development, and lobbying, the (Bayes) belief updating procedure is not one-shot, and agents’ optimal signaling strategies rely on the responses of sequentially updated beliefs. This demands approaches to systematically analyze higher-order inference of agents and derive each agent’s optimal manipulation of information revelation to serve her own objective. However, since the cardinality of the information space grows exponentially with the order of inference, approaches that serve both purposes can be complicated and hard to analyze. In order to advance the theoretical understanding of higher-order inference in games with communications, this thesis studies several models in social learning and information design to derive approaches to solve particular questions. Our results in social learning models present an elegant approach to accumulate information under higher-order inference among agents, and our results in information design highlight (1) the fragility of optimal signaling strategies under higher-order inference; and (2) the significance of the order of commitment in information design problems.
Chair: Professor Vijay Subramanian