Communications and Signal Processing Seminar
Steins Method for Big-Data Systems: From Learning Queues to Q-Learning
Add to Google Calendar
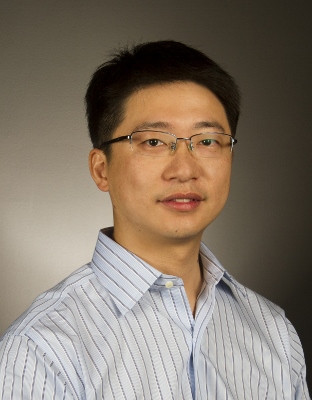
Big-data analytics involves data collection, data processing, and mining of and learning from data. Stochasticity is ubiquitous in big-data analytics, and often one of the main obstacles in the design, control and analysis of big-data systems. In the first part of this talk, I will review some open problems in private-data market design, cloud computing and reinforcement learning related to stochastic analysis. I will then introduce an analytical framework, inspired by Stein's method in probability theory, for analyzing stochastic big-data systems. I will present two previously open problems which we solved recently: (i) how much information is needed to balance the load in cloud computing systems to achieve asymptotically zero queueing delay? (ii) how many samples are needed in reinforcement learning to learn value functions or Q-functions with function approximation?
Lei Ying received his Ph.D in Electrical and Computer Engineering from the University of Illinois at Urbana-Champaign. He currently is a Professor at the School of Electrical, Computer and Energy Engineering at Arizona State University. His research is broadly in the interplay of complex stochastic systems and big-data, including large-scale communication/computing systems for big-data processing, private data marketplaces, and large-scale graph mining. He won the Young Investigator Award from the Defense Threat Reduction Agency (DTRA) in 2009 and NSF CAREER Award in 2010. His research contributions have been recognized as best papers in conferences across different disciplines, including communication networks (INFOCOM andWiOpt), computer systems (SIGMETRICS) and data mining (KDD).