Communications and Signal Processing Seminar
Probability on graphs: Centrality, Optimization, and Social Learning
Add to Google Calendar
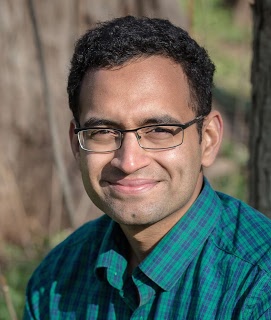
Recent years have seen a proliferation of large datasets possessing some type of network structure. In this talk, we shall discuss three separate problems that lie at the intersection of network science, probability, and combinatorics. In the first problem, we study a phenomenon called "persistence of centrality" in growing random graphs models such as preferential attachment and uniform attachment trees. In the second problem, we study function optimization on a graph. Here, the goal is to find a vertex where a graph function is maximized using local iterative algorithms that are inspired by gradient descent and related algorithms in continuous optimization. Finally, our third problem discusses a simple model for social learning involving a two vertex graph with a "teacher" and a "student." We show that such a model leads to a counterintuitive phenomenon where a student learns better when the teacher is worse. Our three problems demonstrate the impact of probabilistic tools on a variety of network science problems.
Varun Jog received his B.Tech. degree in Electrical Engineering from IIT Bombay in 2010, and his Ph.D. in Electrical Engineering and Computer Sciences (EECS) from UC Berkeley in 2015. Since 2016, he is an Assistant Professor at the Electrical and Computer Engineering Department and a fellow at the Grainger Institute for Engineering at the University of Wisconsin – Madison. His research interests include information theory, machine learning, and network science. He is a recipient of the Eli Jury award from the EECS Department at UC Berkeley (2015) and the Jack Keil Wolf student paper award at ISIT 2015.