Faculty Candidate Seminar
Optimization and Learning in Energy Systems: Privacy and Performance
Add to Google Calendar
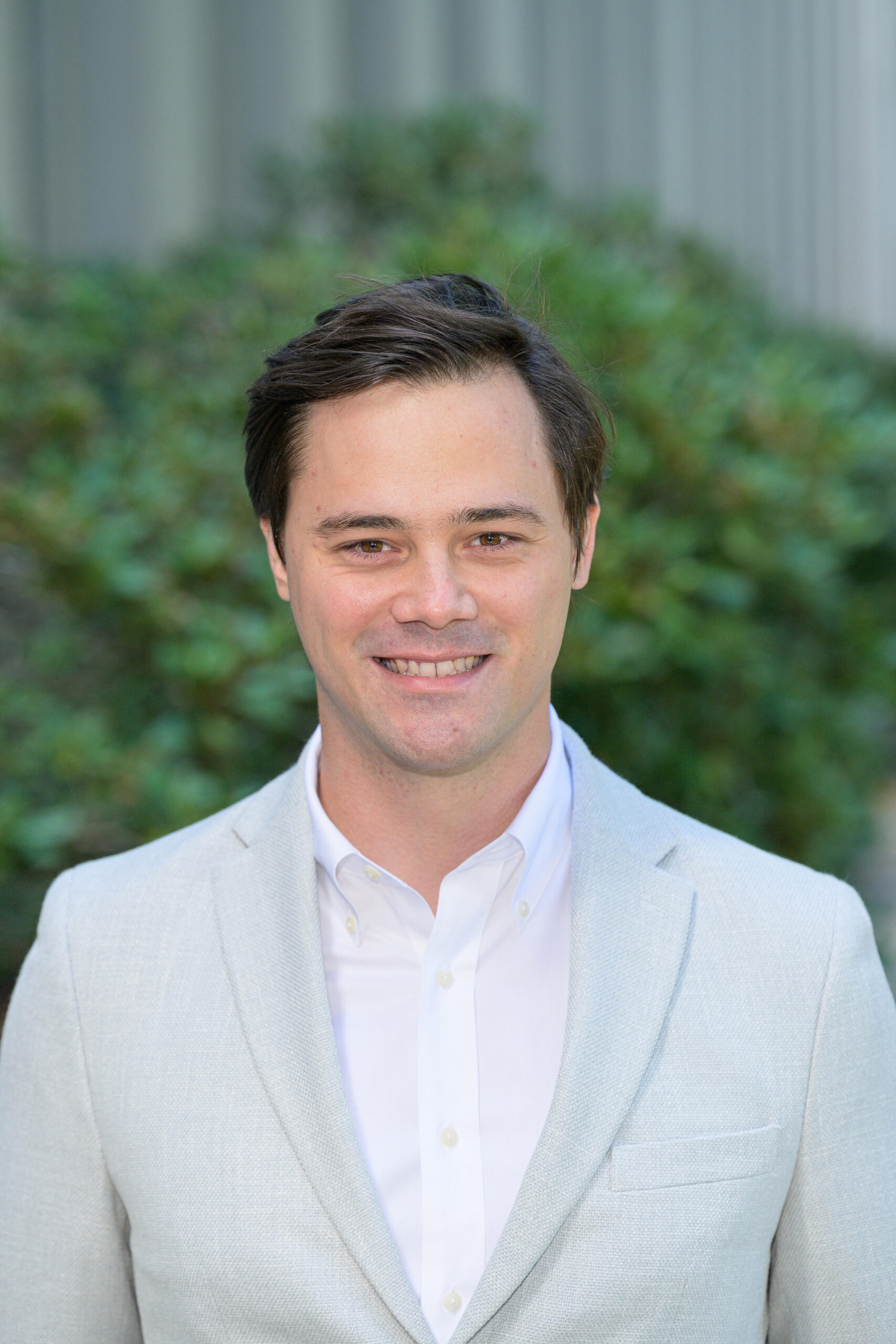
Zoom Link: Password for remote participants is ECECAN
Abstract: Stochastic programming and machine learning are transformative decision-making technologies that enable rapid integration of intelligent metering and control devices and motivate the use of data-centric algorithms in renewable-dominant energy systems. Implementing these technologies in practice, however, is obstructed by the following two major barriers: (a) mistrust towards model-agnostic optimization and machine learning, and (b) hesitance to share data (time series, network topology, market bids, etc.) due to the risks of exposing private and critical information. This talk describes how stochastic programming, deep learning, and data science, taken together, unlock the development of trustworthy and privacy-preserving decision support and pricing under uncertainty for various applications in energy systems and markets. The talk first explains performance guarantees (e.g., feasibility and optimality) in a stochastic network control/design problem, and then how to enhance solutions to this problem by augmenting trained deep learning models in a computationally tractable manner. The next part details the nexus of stochastic programming and algorithmic (differential) privacy to establish rigorous privacy guarantees for secure data utilization and sharing in the energy sector.
Bio: Vladimir Dvorkin is a postdoctoral fellow at the Massachusetts Institute of Technology (MIT) affiliated with the Energy Initiative and Laboratory for Information & Decision Systems. Before joining MIT, he earned a PhD degree in electrical engineering at the Technical University of Denmark from 2017 to 2021, under the supervision of Prof. Pierre Pinson and Prof. Jalal Kazempour, and was a visiting scholar at the School of Industrial and Systems Engineering of Georgia Tech, under the supervision of Prof. Pascal Van Hentenryck. In his research, Dvorkin has worked on energy transition through the lens of decision-making under uncertainty, energy economics, and algorithmic privacy. Today, he broadens this portfolio towards certifying decisions under uncertainty, providing algorithmic privacy to arbitrary optimization datasets, and developing optimization algorithms to assist machine learning tasks in energy. His work is recognized by several prestigious awards, including the Marie Skłodowska-Curie Actions & Iberdrola Group postdoctoral fellowship and the IEEE Transactions on Power Systems Best Paper Award. Dvorkin is a co-founder and co-organizer of the “enOPTIMAL: Energy, Optimization and Learning” seminar series at MIT.