Dissertation Defense
Improving Articulated Pose Tracking and Contact Force Estimation for Qualitative Assessment of Human Actions
This event is free and open to the publicAdd to Google Calendar
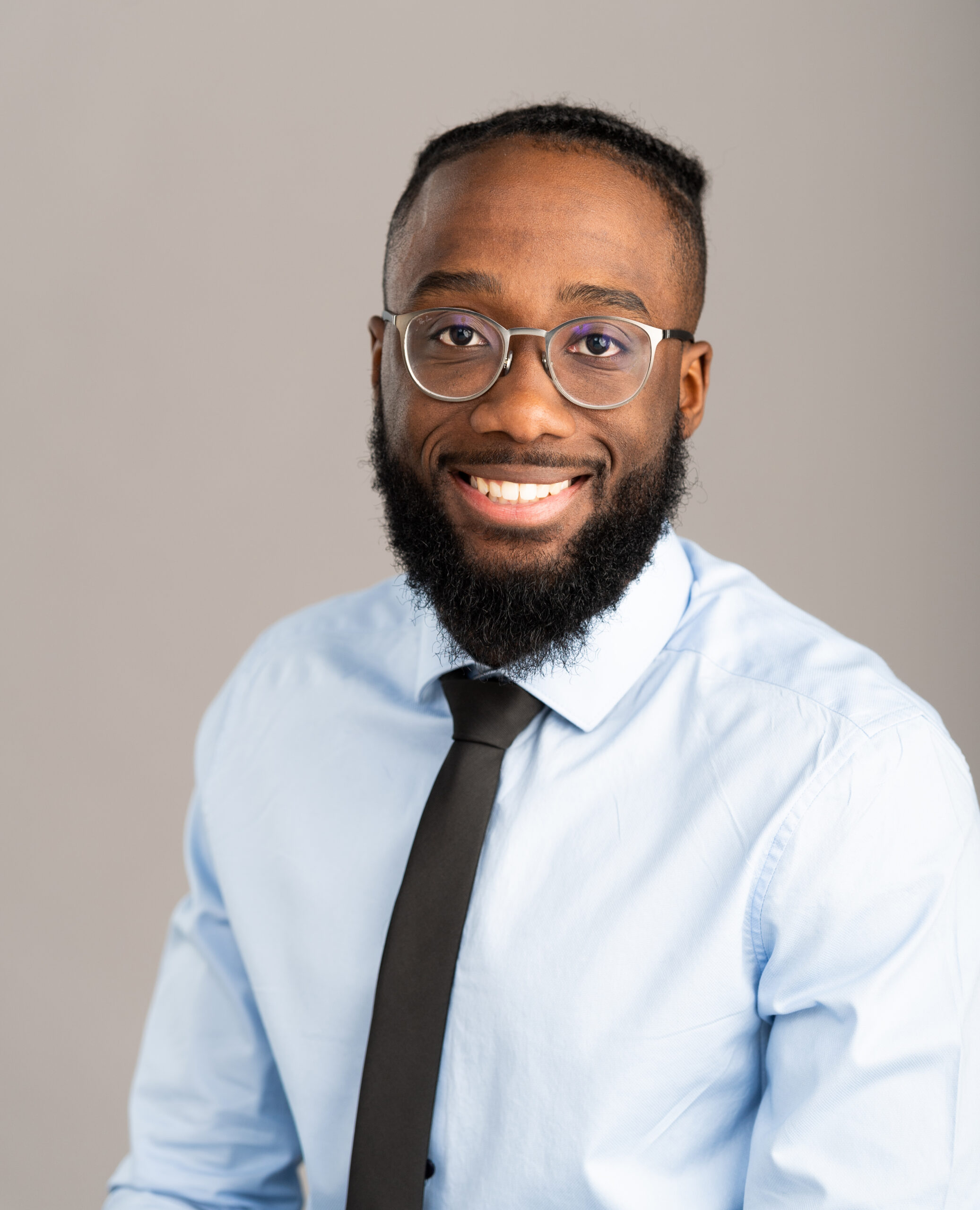
PASSCODE: vision
Using video for automated human performance metrics or skill analysis is a crucial yet underexplored task. While expert assessment can be subjective and biased, computer vision and AI offer real-time, objective, and scalable solutions across various domains. From video alone, we can automatically score Olympic sports, evaluate the technical skill of surgeons for training, or monitor the physical rehabilitation progress of a patient.
This dissertation focuses on hand and full body poses as the bases for human analysis, starting with proposed methods to enhance articulated hand pose tracking accuracy in the surgical domain. We then demonstrate improved surgical skill assessment performance through contrastive pre-training on embedded hand features. For human poses, we bridge the gap between visual observations and the physical world by estimating ground contact forces, utilizing multitask learning to integrate motion capture data without additional force plate supervision. To further enhance physical understanding, we introduce novel simulation-based metrics for evaluating the physical plausibility of human poses. Finally, to mitigate data limitations, we introduce two new datasets: SurgicalHands, a complex hand pose tracking dataset to improve surgical applications, and ForcePose, a comprehensive GRF dataset enabling physical grounding of motion.
CHAIR: Professor Jason J. Corso